The Essential Role of Scalable Databases in AI-Powered Applications
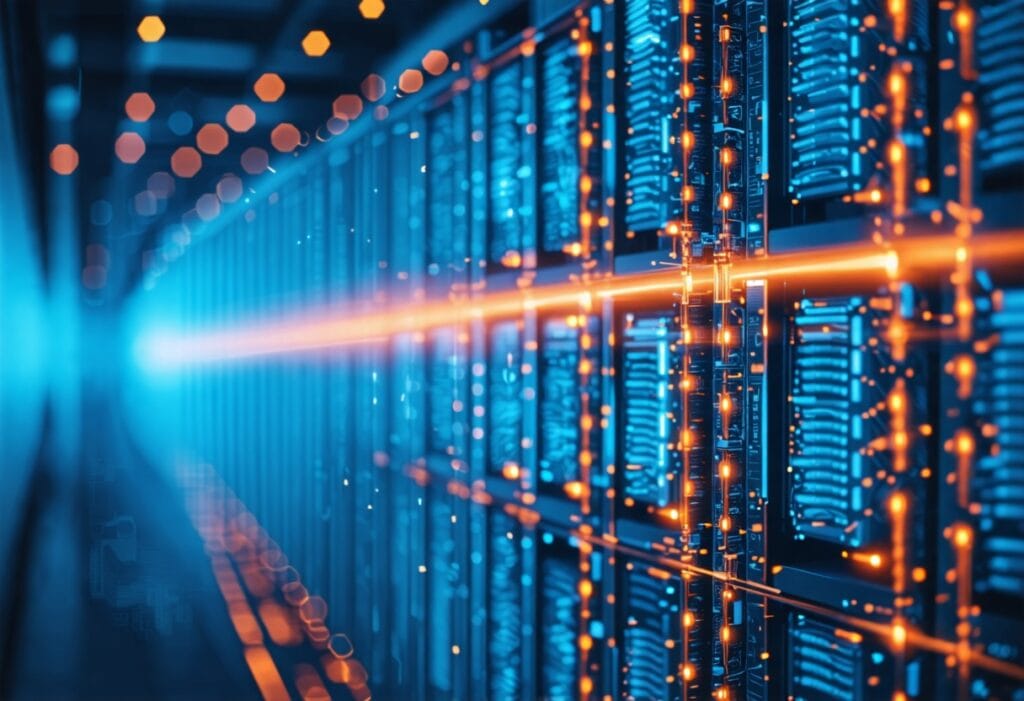
The Role of Scalable Databases in AI-Powered Applications
In today’s rapidly evolving technological landscape, the integration of artificial intelligence (AI) into applications is transforming how businesses operate, making scalable databases more crucial than ever. As AI continues to grow in complexity, the ability to manage and analyze vast amounts of data in real-time has become a paramount challenge. Scalable databases emerge as a vital solution, enabling organizations to support generative AI functionalities that drive efficiency and innovation. With the predictive capabilities and adaptive optimization that AI offers, businesses can enhance performance and resource allocation, significantly improving operational efficiency while reducing costs.
This article delves into the intersection of scalable database technology and AI, highlighting how these powerful tools can meet the demanding needs of modern applications. Whether addressing the challenges of processing diverse data types or ensuring swift data retrieval for AI model training, understanding the importance of a robust database framework is essential for current and future AI-powered applications. Join us as we explore key considerations for selecting the right scalable database and the common pitfalls organizations face when integrating AI into their operations.
Understanding the Importance of Scalable Databases in AI
As organizations recognize the power of artificial intelligence in driving business solutions, the need for scalable databases has grown exponentially. Scalable databases serve as the backbone for AI applications, providing the necessary infrastructure to handle increasing data volumes. This section explores the fundamental role these databases play in enabling effective AI operations.
Real-Time Data Processing
One of the primary requirements for effective AI applications is the ability to process and analyze data in real-time. Traditional databases often struggle to maintain the necessary speeds when faced with high-velocity incoming data streams. Scalable databases, equipped with horizontal scalability and partitioning capabilities, can manage extensive datasets across multiple nodes, ensuring that AI applications can respond to queries rapidly and accurately. This speed is critical for applications relying on machine learning for real-time inference, such as fraud detection and personalized recommendations.
Diverse Data Management
AI applications often need to work with a plethora of data types—structured, unstructured, and semi-structured data. Scalable databases are designed to handle this variety seamlessly. They enable businesses to store everything from JSON documents to massive unstructured media files without requiring extensive extraction, transformation, and loading (ETL) procedures. This capability allows organizations to rapidly integrate new data sources into their AI models, enhancing the richness and quality of the insights generated.
The Challenges Facing Traditional Databases
Although scalable databases offer many advantages, organizations face significant challenges when relying on traditional systems for modern AI applications. This section delves into the limitations of conventional databases that drive the need for scalable solutions.
Performance Bottlenecks
Traditional databases often encounter performance bottlenecks as data demands increase. As AI applications require continuous data ingestion and processing, conventional databases may struggle to meet these requirements, leading to latency issues. This is particularly evident in applications that depend on fast querying to provide real-time intelligence. In contrast, scalable databases can dynamically allocate resources based on current demand, significantly reducing bottlenecks.
Management Complexity
Integrating AI into existing workflows often introduces added complexity, especially when traditional databases are involved. The need for continuous updates and model retraining increases the challenge of managing data flows effectively. Scalable databases facilitate this integration by enabling more straightforward data management practices. Their architecture allows for easier experimentation and iteration cycles, crucial for developing AI solutions that adapt to changing inputs quickly.
Key Features of Scalable Databases for AI Applications
When selecting a scalable database to support AI functionality, businesses should prioritize certain features that enhance their operational capabilities. This section highlights the essential traits to consider.
Horizontal Scalability
Horizontal scalability is perhaps the most critical feature for scalable databases, allowing organizations to add additional servers or nodes to accommodate increased data loads. This approach provides elasticity in data management and ensures that the database can grow alongside the demands of the AI models. For organizations employing AI across multiple departments, horizontal scalability allows for the seamless expansion of resources without the need for complete system overhauls.
Integration with AI and Data Science Tools
Organizations should consider databases that offer native support for data science and AI tools. Integration capabilities with popular frameworks like TensorFlow or PyTorch can streamline workflow processes and accelerate the deployment of AI models in production. Additionally, having a database that seamlessly connects with existing data science and analytics tools allows teams to work efficiently, maximizing productivity while developing and iterating on AI-driven features.
Real-World Applications of Scalable Databases in AI
Understanding the theoretical aspects of scalable databases is crucial, but real-world applications can provide deeper insights into their practical benefits. This section explores diverse sectors where scalable databases significantly enhance AI functionalities.
Healthcare Analytics
In the healthcare sector, AI applications are increasingly being employed for predictive analytics, patient management, and clinical decision support. Scalable databases enable healthcare organizations to analyze extensive datasets from various sources, including patient records, lab results, and real-time monitoring systems. This ability helps medical professionals make informed decisions and enhances patient outcomes by ensuring immediate access to critical data.
Retail and E-Commerce
Retailers leverage AI algorithms to improve customer experiences, manage inventory more efficiently, and optimize supply chains. Scalable databases facilitate the real-time processing of consumer data, allowing businesses to personalize marketing strategies and anticipate purchasing trends. For example, e-commerce companies can utilize predictive analysis to manage stock levels effectively, ensuring that supply meets demand without incurring unnecessary costs.
Financial Services
The financial services industry continuously seeks ways to improve risk management, fraud detection, and customer service applications. By utilizing scalable databases, organizations can perform advanced analytics on extensive transactional datasets, enabling real-time monitoring and alert systems to identify fraudulent activities. Moreover, tailored AI models can personalize banking experiences, enhancing customer engagement and satisfaction.
Simplifying the Adoption of AI with Databases
As organizations navigate the complexities of AI adoption, scalable databases emerge as crucial enablers of success. This section discusses how these databases simplify the implementation of AI technologies.
Quick Deployment and Iteration
With scalable databases, businesses can rapidly deploy AI applications without extensive preliminary setups. The ability to handle fluctuating demands and accommodate a wide array of data types means that organizations can iterate on their AI models more quickly, fostering a culture of innovation. This rapid iteration capability is vital in dynamic environments where market conditions or consumer preferences can change abruptly.
Resource Optimization
The adaptive nature of scalable databases ensures that organizations can optimize their resources efficiently. By providing robust performance without overprovisioning hardware, businesses can reduce costs related to infrastructure maintenance and management. This financial efficiency is particularly appealing for startups and smaller enterprises that rely on limited resources to push their AI initiatives forward.
Future Trends in Scalable Databases for AI
As the landscape of artificial intelligence continues to evolve, scalable databases will play an increasingly pivotal role in shaping future developments. This section outlines potential trends and their implications for businesses.
Increased Automation of Database Management
The rise of AI-driven solutions will lead to the automation of various database management tasks, such as scaling, data migration, and performance tuning. This shift will allow organizations to focus their resources on higher-value tasks while enhancing operational efficiency.
Enhanced Security Measures
As data privacy and protection become more crucial, scalable databases are likely to adopt advanced security measures. These measures will ensure that sensitive data is protected while still allowing for analytical processing, enabling businesses to meet regulatory requirements without sacrificing accessibility.
Integration with Emerging Technologies
Looking ahead, scalable databases will increasingly integrate with emerging technologies such as blockchain and IoT. This convergence will provide organizations with a holistic approach to data management, enhancing AI models’ capabilities and broadening the scope of applications across various industries.
Unlocking the Future of AI with Scalable Databases
In summary, scalable databases are not just an asset but a necessity for businesses aiming to harness the full potential of AI-powered applications. They address critical challenges associated with data processing, integration, and management, ensuring organizations can operate efficiently in a competitive landscape. As AI technologies evolve, the importance of having a robust database infrastructure will only increase, enabling real-time insights and enhancing operational capabilities across a variety of sectors.
By prioritizing key features such as horizontal scalability, seamless integration with AI tools, and adaptability to diverse data types, organizations can position themselves at the forefront of innovation. Embracing scalable databases allows companies to streamline their AI initiatives, optimize resources, and respond quickly to market demands. As we look toward the future, staying informed about emerging trends and technologies in database management will be crucial for any business seeking to thrive in the AI era.
Investing in scalable database solutions today will lay the groundwork for successful AI integration tomorrow, driving sustained growth, enhanced customer experiences, and improved decision-making capabilities.