Revolutionizing Robot Training with Heterogeneous Pretrained Transformers
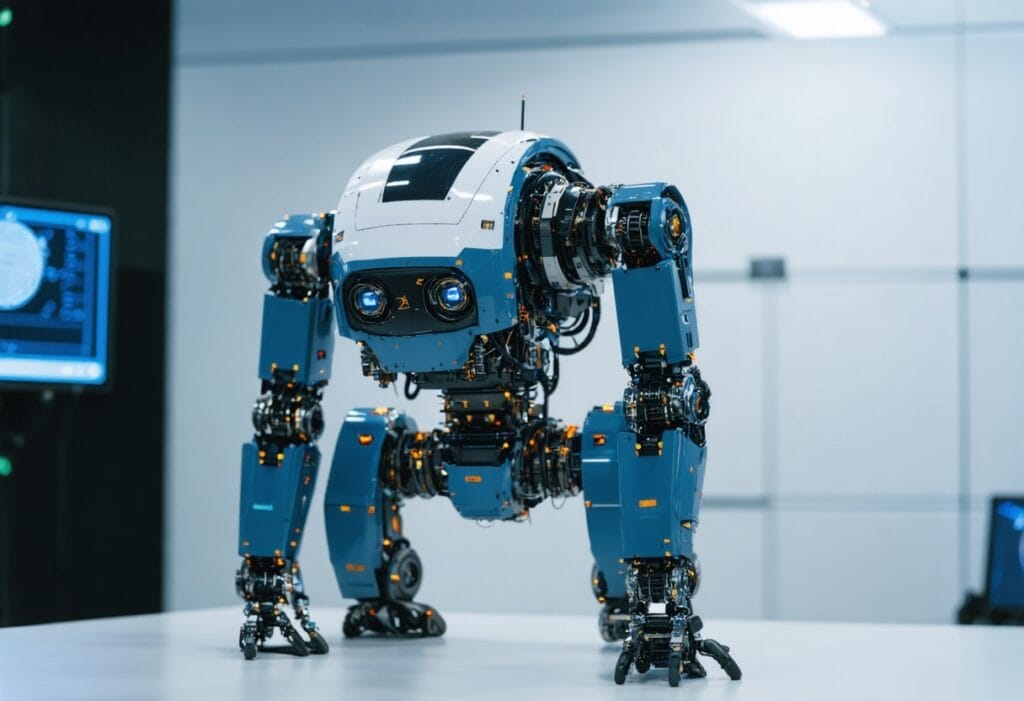
Revolutionizing Robot Training: An MIT Breakthrough
In the realm of robotics, efficient training methods are essential for enabling robots to adapt to diverse tasks and environments. A recent breakthrough at MIT introduces a transformative approach known as Heterogeneous Pretrained Transformers (HPT), which promises to significantly reduce both time and costs associated with robot training while enhancing adaptability. This innovative methodology consolidates vast amounts of diverse data from multiple sources into a cohesive system, paving the way for robots to understand and perform tasks with unprecedented agility.
Traditional robot training often involves collecting specific datasets for individual robots, a process that can be both labor-intensive and limited in scope. However, the MIT team’s novel architecture unifies various data types—including visual inputs, language instructions, and proprioceptive information—creating a ‘shared language’ that generative AI models can adeptly process. With impressive real-world applications demonstrating more than a 20 percent improvement over conventional training techniques, this development is set to redefine the landscape of robotics.
As researchers continue to explore the full potential of HPT—including its capability to process unlabelled data—the exciting prospect of a universal robot brain draws nearer. This article delves deeper into the mechanics of this groundbreaking innovation and its implications for the future of robotic training and functionality. Stay tuned to discover how MIT is shaping the next generation of intelligent machines.
Understanding Heterogeneous Pretrained Transformers (HPT)
The Heterogeneous Pretrained Transformers (HPT) methodology, developed at MIT, represents a significant advancement in the way robots are trained. Traditional robot training regimens typically focus on single modalities—such as visual data or specific task instructions—which can limit the versatility and adaptability of the robotic systems. HPT, on the other hand, integrates multiple data types into a single framework, allowing robots to interpret and respond to a broader range of inputs. This is critical for tasks that require real-time processing of different forms of information.
Central to the HPT approach is the use of transformer models, which are already widely used in natural language processing. These models excel at understanding context and bridging various types of information, making them ideal for the complex demands of robotic training. By utilizing the strengths of transformers, HPT can not only decrease training times but also equip robots with an enhanced ability to navigate tasks that they have not been explicitly trained on.
The Data Revolution: Unifying Diverse Inputs
One of the key innovations of HPT is its capacity to unify disparate data sources. The architecture developed by the MIT team can process visual images, language instructions, and proprioceptive data—information regarding the robot’s own movements and positioning. This multilayered approach facilitates a comprehensive understanding of tasks, enabling more nuanced actions in complex environments.
The team amassed an extensive dataset comprised of 52 individual datasets and over 200,000 robot trajectories. This rich repository of experiences mirrors human learning, where exposure to a variety of scenarios enhances understanding and adaptability. This approach not only improves the robot’s current capabilities but also lays a robust foundation for future learning as it encounters new challenges.
Real-World Applications and Testing Results
Testing the effectiveness of HPT in both simulated and real-world scenarios has yielded impressive results. Robots using the HPT methodology outperformed those trained using conventional methods by more than 20%. This success underscores the system’s ability to generalize knowledge from a wide range of tasks rather than relying on a narrow set of training data.
The implications of these findings extend beyond merely saving time and costs in robot training. The enhanced adaptability also suggests that robots could be deployed in more dynamic environments, such as homes, hospitals, or disaster zones, where rapid task switching and real-time decision-making are essential. The ability to adapt quickly without extensive retraining sessions represents a leap forward in practical robotics applications.
The Role of Proprioception in Advanced Robotics
Another notable aspect of HPT is its innovative approach to proprioception. Historically, many robotic training models have prioritized visual input over proprioceptive awareness, often leading to less dexterous and less capable robotic movements. The MIT team’s architecture addresses this by equally weighing the importance of proprioceptive data alongside visual cues, resulting in robots that can execute more intricate motions.
For instance, tasks requiring delicate manipulations, similar to those performed by human hands, can now be achieved with greater precision. Robots that leverage this enhanced proprioceptive awareness can improve their interactions with physical objects, making them more effective in various sectors such as manufacturing, healthcare, and personal assistance.
A Future with Universal Robot Intelligence
The research team at MIT is ambitious in its vision for the future, aspiring to enhance HPT’s capabilities further by processing unlabelled data, akin to how advanced language models function. This opens the door to the concept of a universal robot brain—an intelligence framework that any robot could utilize without additional customization or training. This would drastically reduce the overhead costs and timescales required to deploy robotic solutions in various contexts.
As researchers enhance HPT’s ability to understand context from unlabelled data, the potential for breakthroughs in robotic functions expands significantly. If successful, this could lead to robots that effortlessly learn and adapt to their environments much like humans do, rather than relying solely on pre-programmed instructions.
Challenges and Future Directions
Despite the remarkable progress made, the MIT team acknowledges that they operate in the early stages of developing the HPT model. Several challenges remain, particularly in scaling the system for broader applications and refining its learning mechanisms to handle the complexity of real-world tasks.
Ongoing research is geared towards improving the model’s efficiency and capability in assimilating diverse inputs in practical settings. As HPT and similar architectures evolve, they have the potential to revolutionize industries by making robotic systems more intelligent, autonomous, and capable of engaging in complex, multi-faceted tasks without the constraints of traditional training methodologies.
Conclusion
The implications of the HPT breakthrough are broad and transformative. With the ability to integrate diverse data types and enhance robot adaptability, MIT’s innovation could set the stage for the next generation of intelligent robotics, paving the way for smarter robots capable of navigating the complexities of the modern world. As the research progresses, the vision of a versatile, universally applicable robotic intelligence may soon become a reality.
Embracing the Future of Robotics with HPT
As robotics continues to advance at a rapid pace, the introduction of Heterogeneous Pretrained Transformers (HPT) represents a pivotal moment in the field. By effectively merging diverse data inputs into a cohesive learning framework, this innovative approach is set to enhance the adaptability and efficiency of robotic systems dramatically. The achievements demonstrated through HPT not only promise significant reductions in training time and costs but also open new avenues for deploying robots in increasingly complex environments.
With real-world applications proving a substantial performance boost, HPT positions robots to perform dynamic tasks in various sectors—from healthcare to emergency response—where swift adaptability is crucial. Furthermore, the emphasis on proprioceptive awareness lends a new dimension to robotic dexterity, making interactions more natural and effective.
As researchers diligently refine the HPT model, the vision of a universal robotic intelligence is on the horizon, potentially transforming how robots learn and operate in an ever-evolving landscape. The implications of this breakthrough extend well beyond technical advancements, heralding a future where intelligent machines seamlessly integrate into daily life, enhancing productivity and enriching the human experience. The journey toward fully autonomous and adaptable robotics is just beginning, and the potential is limitless.