Enhancing AI Safety Through Innovative Red Teaming Approaches
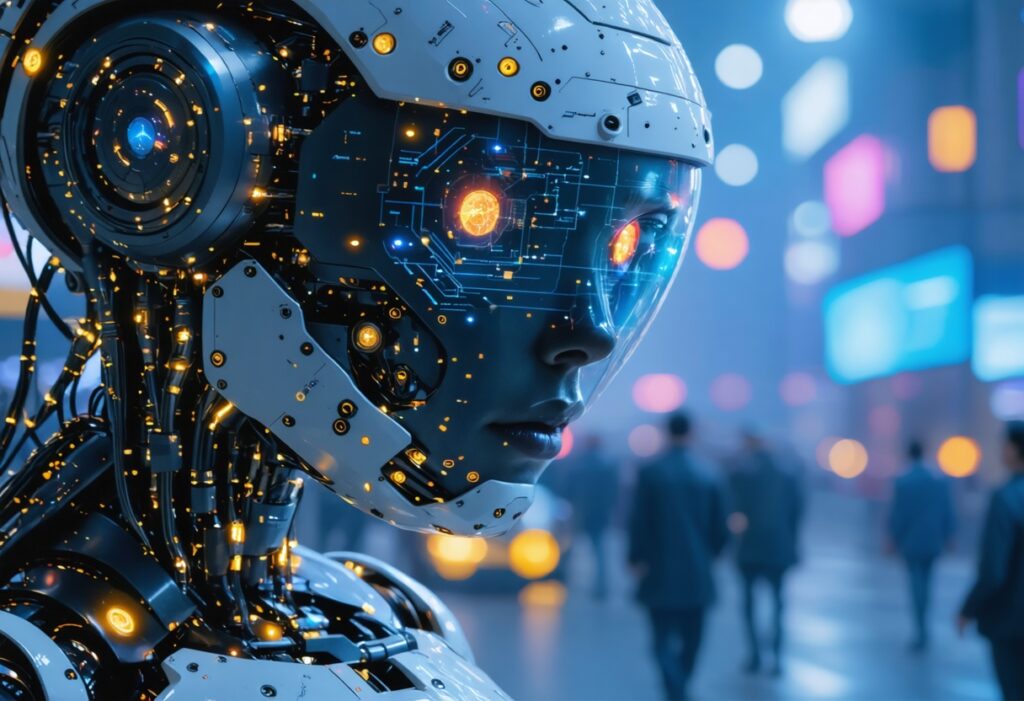
Enhancing AI Safety: OpenAI’s Innovative Red Teaming Approaches
As artificial intelligence technology rapidly evolves, ensuring its safety and ethical use has become a paramount concern for developers and researchers alike. OpenAI is at the forefront of this effort, implementing advanced red teaming methodologies to uncover potential risks within AI systems. Red teaming, a structured approach that engages both human experts and AI tools, allows OpenAI to proactively identify vulnerabilities and ensure robust safeguards are established. Historically reliant on manual testing, OpenAI is now integrating automated processes in its red teaming strategy, drastically improving the efficiency and effectiveness of risk assessments.
This article delves into OpenAI’s latest advancements in red teaming, highlighting their innovative techniques for enhancing safety measures and the significance of external collaborations. By sharing insights from recent research documents focused on automated red teaming and external engagement strategies, OpenAI aims to foster a more secure landscape for AI development. As the conversation surrounding AI ethics and safety grows, understanding these new methodologies becomes crucial for stakeholders committed to responsible AI governance.
Understanding OpenAI’s Red Teaming Methodology
Red teaming is an essential strategy employed by OpenAI to identify and mitigate risks associated with AI models. Unlike traditional forms of testing that might rely heavily on manual input, OpenAI’s approach efficiently combines human oversight with powerful AI capabilities. This dual strategy not only accelerates the identification of potential weaknesses within AI systems but also enriches the overall assessment through a well-rounded perspective.
The methodology for effective red teaming has evolved over time. In the past, the focus was predominantly on human-led assessments where specified experts challenged AI systems to uncover vulnerabilities. However, the recent shift towards integrating automated systems allows for a more scalable and systematic approach. OpenAI’s innovative incorporation of automated processes means that potential flaws can be examined at an unprecedented scale, ensuring the systems can withstand a variety of real-world scenarios.
The Four Pillars of Effective Red Teaming
OpenAI has developed a robust framework consisting of four fundamental steps crucial for conducting red teaming campaigns effectively:
- Composition of Red Teams: The selection process ensures that team members possess a diverse range of expertise. Team members come from varied backgrounds—spanning cybersecurity, social sciences, and policy—to address the multifaceted nature of potential risks.
- Access to Model Versions: To ensure thorough testing, it is essential to determine which model versions are accessible to red teamers. Different stages of model development can reveal unique vulnerabilities, and understanding these variances is critical in building resilient AI systems.
- Guidance and Documentation: Clear instructions and structured documentation are vital for the success of red teaming efforts. Providing comprehensive descriptions of the AI models and existing safeguards ensures that red teamers can effectively test and evaluate their findings.
- Data Synthesis and Evaluation: Once campaigns are wrapped up, teams meticulously analyze the collected data. This analysis helps identify whether findings align with existing safety policies or whether new measures need to be developed. This aligns with the ongoing evolution of safety protocols in AI development.
Real-World Applications of Red Teaming
One notable application of these red teaming processes is the thorough preparation of the OpenAI o1 model family for public deployment. By subjecting these models to rigorous testing against potential misuse scenarios, the team can gauge how effectively they can prevent harm in various applications—ranging from malicious uses to real-world risk assessments. This proactive stance ensures that AI tools released to the public come equipped with robust safety measures and mitigations.
The multi-disciplinary approach lies at the heart of effectively addressing emerging challenges in AI governance. Engaging with experts who understand the nuances of societal implications fosters a comprehensive framework that guides the development of AI technologies. As these technologies are increasingly incorporated into everyday life, holistic assessments become ever more critical.
Automated Red Teaming Techniques
OpenAI has been pioneering various automated processes in its red teaming efforts, demonstrating an ability to quickly generate potential use-case scenarios. This new method facilitates large-scale evaluation without the limitations of traditional manual testing approaches. One compelling technique currently being explored is called “Diverse And Effective Red Teaming With Auto-Generated Rewards And Multi-Step Reinforcement Learning.” This approach aims to promote diversity in testing scenarios, enhancing the robustness of AI applications against unforeseen exploits.
By generating distinct scenarios—such as cases where AI might suggest harmful or unethical actions—OpenAI can critically assess how its models respond in varied contexts. This method emphasizes rewarding diverse outcomes, encouraging the system to recognize and address faults across an expansive spectrum.
Challenges and Limitations of Red Teaming
Despite the progressive strides in red teaming methodologies, challenges persist. OpenAI acknowledges that these techniques capture risks at specific points in time, which may not accurately reflect the broad spectrum of evolving AI capabilities and potential misuse. As AI models develop, so do the threats and vulnerabilities associated with them, necessitating ongoing assessments and updates to testing methodologies.
Another concern revolves around the potential for red teaming activities to create information hazards. By uncovering vulnerabilities in a systematic way, there is a risk of inadvertently revealing these weaknesses to malicious entities. Such risks underline the importance of establishing robust protocols and responsible disclosure practices within the red teaming framework.
The Future of AI Safety Through External Collaboration
In conjunction with rigorous internal practices, OpenAI actively promotes the importance of external collaboration in red teaming efforts. Engaging with outside experts opens channels for fresh perspectives and diverse insights necessary for a holistic understanding of AI risks. Such collaborations enable the identification of the broader societal implications of AI usage, guiding developers towards acceptable practices that align technology with societal values.
As discussions surrounding AI safety gain momentum, OpenAI’s commitment to transparency and public engagement via red teaming initiatives is more critical than ever. Ongoing outreach and collaboration help shape the future framework for AI governance, ensuring that the technology serves humanity effectively and ethically. This dialogue plays a vital role in understanding the diverse viewpoints surrounding the responsibilities of AI developers and users alike.
Embracing the Evolution of AI Safety
As the landscape of artificial intelligence continues to shift, the role of red teaming as a cornerstone for ensuring safety and ethical considerations becomes increasingly vital. OpenAI’s commitment to innovative red teaming methodologies not only enhances the resilience of AI models but also cultivates a culture of proactive risk management. By integrating automated processes and fostering interdisciplinary collaboration, OpenAI sets a benchmark for responsible AI governance and safety measures.
Looking ahead, the future of AI safety will hinge on continuous improvements in red teaming techniques and the cultivation of external partnerships. Stakeholders across the board must remain engaged in dialogues that advocate for the responsible use of AI, reflecting societal values and ethical considerations. As AI technologies integrate more fully into our daily lives, understanding and enhancing these safety measures is essential for building public trust and ensuring that developments serve a beneficial purpose for all.