Unlocking the Future of Materials Discovery with Microsoft’s MatterGen
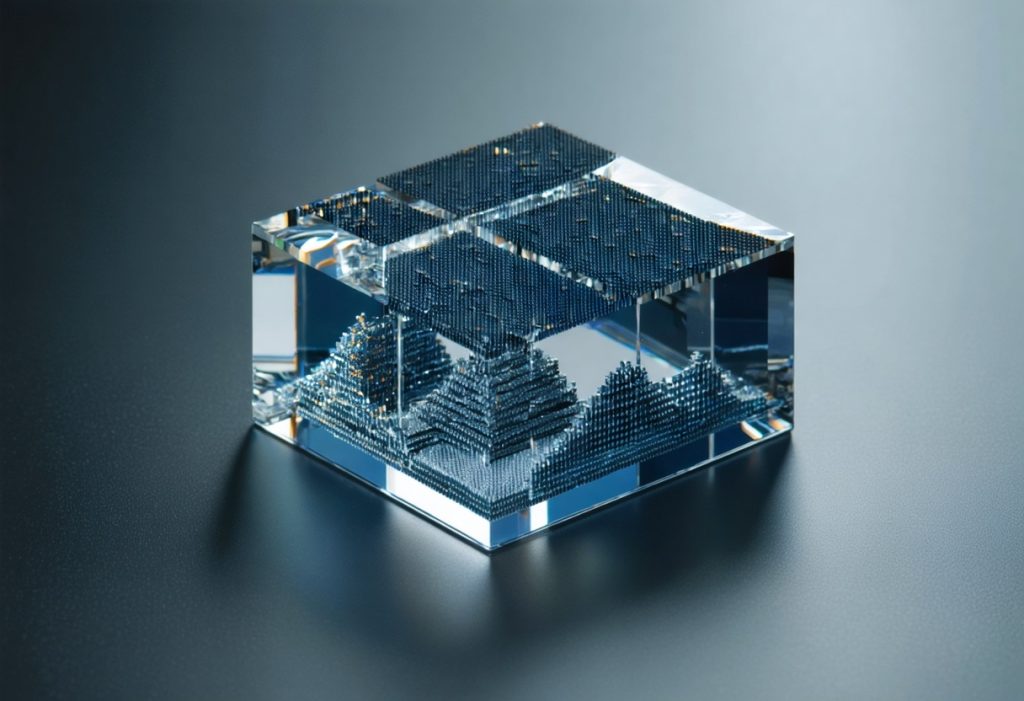
Unlocking the Future of Materials Discovery with Microsoft’s MatterGen
The search for new materials is crucial in addressing some of the most pressing challenges facing humanity today. Traditional methods often resemble “finding a needle in a haystack,” but Microsoft’s innovative generative AI tool, MatterGen, is set to revolutionize this process. By moving beyond conventional computational screening, MatterGen directly engineers novel materials tailored to specific design requirements. This groundbreaking approach promises to significantly enhance the efficiency of materials discovery, facilitating advancements in industries such as renewable energy, electronics, and aerospace engineering. With MatterGen, researchers can explore the vast realm of unknown materials, transforming the landscape of scientific innovation. Discover more about how AI is reshaping materials science and follow the latest developments in this exciting field.
For more insights into the intersection of AI and materials discovery, check out this comprehensive study.
How MatterGen Revolutionizes Material Discovery
The advent of generative AI tools promises to significantly change the landscape of materials discovery. Prior to the release of Microsoft’s MatterGen, discovering new materials was a complex process fraught with inefficiencies. Traditional methods often required extensive computational resources to screen numerous potential candidates against desired physical properties, leading to lengthy research timelines. However, MatterGen introduces a paradigm shift by directly engineering novel materials tailored to specific design requirements, transforming how researchers approach this pivotal field.
The Mechanics of MatterGen
At its core, MatterGen operates as a diffusion model within the 3D geometry of materials. Unlike typical image generation models that alter pixel values based on textual prompts, MatterGen generates complete material structures by manipulating elemental arrangements, positions, and periodic lattices. This bespoke architecture caters specifically to the unique challenges of materials science, such as periodicity and 3D arrangements. Microsoft’s innovative approach enables users to create materials with predetermined chemical compositions and mechanical properties, facilitating unprecedented levels of customization and specificity in materials development.
Moving Beyond Traditional Screening Methods
Traditional computational screening relies on existing databases and historical data to identify candidates for desired properties. While useful, this method has its limitations; researchers often find themselves sifting through millions of existing materials, resulting in diminished returns as the pool of known candidates becomes exhausted. MatterGen, conversely, begins anew with each prompt, producing novel materials based on specific requirements such as mechanical strength, electronic characteristics, or magnetic behavior.
The model has been trained using over 608,000 stable materials sourced from reputable databases like the Materials Project and Alexandria, which enhances its capability to generate unique and novel combinations. This innovative method not only streamlines the discovery process but also opens doors to previously unexplored material possibilities.
Addressing Compositional Disorder in Materials
One common challenge in developing new materials is compositional disorder, where atoms may swap positions within a crystal lattice unexpectedly. Traditional algorithms, which often overlook this variability, frequently conflate similar structures, leading to less effective material designs. To tackle this issue, Microsoft implemented a new structure-matching algorithm within MatterGen that incorporates compositional disorder into its evaluations, resulting in a more robust and accurate definition of novelty in materials synthesis.
Real-World Validation of MatterGen
The capabilities of MatterGen have been expertly validated through collaboration with researchers at Shenzhen Institutes of Advanced Technology (SIAT), part of the Chinese Academy of Sciences. In this partnership, a material named TaCr₂O₆ was synthesized, generated by MatterGen to achieve a bulk modulus target of 200 GPa. The experiment yielded a modulus of 169 GPa, demonstrating a relative error of just 20%, which is significant from an experimental point of view.
The success of this synthesized material not only illustrates MatterGen’s predictive accuracy but also highlights its potential for effectively guiding the design process across various applications such as energy storage, catalysis, and advanced manufacturing.
Integrating AI into Material Simulations
Microsoft has strategically positioned MatterGen as a complementary tool to its earlier AI model, MatterSim, which focuses on accelerating simulations of material properties. Together, these tools create a synergistic “flywheel” effect, enhancing both the exploration of new materials and the iteration of their properties in iterative loops. This powerful combination is a key component of Microsoft’s vision for the “fifth paradigm of scientific discovery,” wherein AI plays a pivotal role, not just in pattern recognition, but actively guiding experimental processes.
Open-Source Access and Future Prospects
In a significant move towards fostering collaboration and innovation, Microsoft has released the source code for MatterGen under the MIT license. Alongside the code, the training and fine-tuning datasets have been made available, aiming to encourage broader adoption of this transformative technology within the materials science community. By embracing an open-source model, Microsoft is paving the way for researchers and developers to extend the capabilities of MatterGen, potentially unlocking even more applications.
AI in Material Science: A Broad Spectrum of Opportunities
The implications of generative AI tools like MatterGen extend far beyond materials science. Similar to how AI has transformed drug discovery and design, MatterGen stands to reshape multiple industries, particularly in renewable energy, electronics manufacturing, and aerospace engineering. As the quest for sustainable and advanced materials continues, tools like MatterGen will play crucial roles in generating innovative solutions that address the challenges of today and tomorrow. For further reading, check out resources on AI in materials science at reputable platforms such as Nature and ScienceDirect.
Future Innovations on the Horizon
As we look to the future, the continuous evolution of generative AI models like MatterGen holds promise for unlocking the full potential of materials discovery. The dual advantage of accelerated discovery and predictive design could lead to breakthroughs in energy efficiency, component durability, and overall material performance. This innovation trajectory reaffirms the role of AI as an indispensable ally in the ongoing pursuit of scientific and industrial advancements.
Embrace the Future of Materials Discovery Today
Microsoft’s MatterGen heralds a new era in materials discovery, significantly reducing inefficiencies traditionally associated with the search for novel materials. By utilizing generative AI, researchers can now custom-engineer materials with specific properties tailored to various applications, from renewable energy to aerospace engineering. The introduction of innovative algorithms to address compositional disorder further enhances its capability, as seen in real-world validations like the successful synthesis of TaCr₂O₆. As MatterGen integrates with existing AI tools, it not only expedites discovery but also empowers researchers to explore uncharted material possibilities. Don’t miss the chance to delve deeper into how AI is reshaping our approach to material science; explore insights through this comprehensive study. Start harnessing the power of generative AI today to contribute to the future of scientific innovation!