DeepSeek-R1: A New Contender in Advanced AI Reasoning
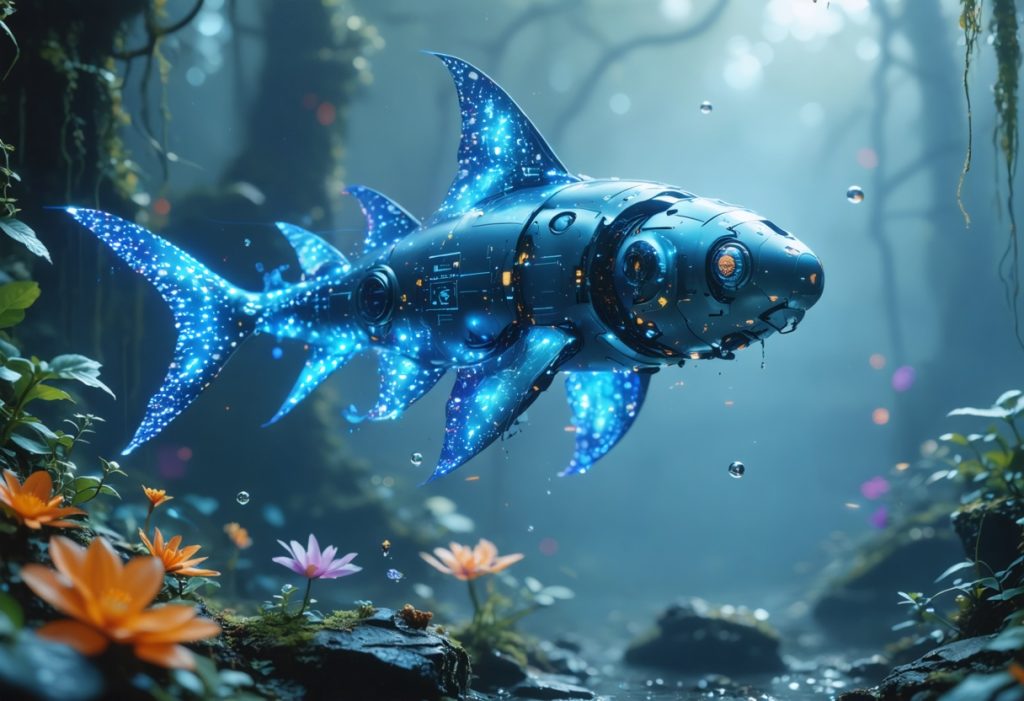
DeepSeek-R1: A New Contender in Advanced AI Reasoning
The realm of artificial intelligence is witnessing an exciting evolution with the introduction of DeepSeek’s latest models, DeepSeek-R1 and DeepSeek-R1-Zero. Designed to excel at complex reasoning tasks, these cutting-edge models are setting new standards in performance, comparable to leading contenders like OpenAI’s renowned systems. DeepSeek-R1, in particular, showcases remarkable advancements by integrating large-scale reinforcement learning, achieving exceptional benchmarks across mathematics, coding, and general reasoning. With promising capabilities that include self-verification and intricate reasoning patterns, DeepSeek is making significant strides in AI, paving the way for innovations that could reshape the industry landscape. Discover more about the implications of these models and their contributions to the open-source movement in AI here.
DeepSeek-R1: An Overview of Its Advanced Reasoning Capabilities
DeepSeek-R1, alongside its variant DeepSeek-R1-Zero, stands at the forefront of AI reasoning models, leveraging state-of-the-art techniques to outperform leading competitors like OpenAI. These models employ innovative methodologies, including large-scale reinforcement learning (RL) and a unique training regimen that eliminates reliance on supervised fine-tuning (SFT). This section delves into the technical aspects of DeepSeek-R1, detailing its advancements and implications for the future of AI.
The Evolution of DeepSeek Models
DeepSeek has embarked on a new journey with its DeepSeek-R1 model, which demonstrates a significant leap in reasoning capabilities. Unlike traditional AI that often depends heavily on SFT, DeepSeek-R1-Zero’s reliance exclusively on RL has resulted in the emergence of complex reasoning behaviors. For a more comprehensive understanding of reinforcement learning’s role in AI, refer to this beginner’s guide to reinforcement learning which outlines its foundational principles.
DeepSeek-R1 improves upon its predecessor by introducing cold-start data during its pre-training phase. This process addresses critical limitations encountered by DeepSeek-R1-Zero, such as endless repetition and poor readability. By integrating cold-start data, DeepSeek-R1 not only enhances reasoning capabilities but also formalizes its position as a formidable competitor against the likes of OpenAI’s renowned models.
Benchmark Performance and Competitive Edge
DeepSeek-R1 has set impressive benchmarks in various tasks, particularly in mathematics, coding, and general reasoning. Remarkably, it achieved a 97.3% score on the MATH-500 benchmark, surpassing OpenAI’s score of 96.4%. Furthermore, its distilled variant, DeepSeek-R1-Distill-Qwen-32B, achieved a notable 57.2% on the LiveCodeBench, showcasing the effectiveness of smaller models. For an in-depth look at benchmarking AI models, consider visiting the Towards Data Science platform, which elucidates various metrics that gauge AI performance.
The Role of Open-Source in AI Advancement
DeepSeek has embraced the open-source philosophy by launching both DeepSeek-R1 and DeepSeek-R1-Zero along with smaller distilled models. This move not only democratizes access to advanced AI technology but also fosters collaboration in the research community. By making these models freely available under the MIT License, DeepSeek encourages innovation and adaptation, creating a ripple effect that could lead to breakthroughs in AI reasoning techniques.
For an exploration of the importance of open source in AI, the OpenAI’s research page can provide insights into similar initiatives and their impact on the broader landscape of artificial intelligence.
Innovative Training Pipeline: A New Approach
DeepSeek’s innovative training pipeline is another factor that distinguishes DeepSeek-R1 from its competitors. The model development process consists of two stages of SFT to build foundational reasoning abilities, followed by two phases of RL tailored for advanced reasoning discovery. This expertly crafted pipeline not only improves model performance but also aligns AI reasoning capabilities with human preferences—a crucial element for real-world applications.
This structured approach allows the development of models that can adapt and refine their reasoning patterns autonomously, marking a pivotal advancement in the field. To understand the broader impacts of structured training in AI models, explore research articles by prominent institutions found at PMC Central.
Challenges Ahead: Addressing Model Limitations
Despite the groundbreaking advancements, DeepSeek-R1 is not without its challenges. Key issues such as endless repetition and language mixing present significant roadblocks that could limit real-world application of the model. DeepSeek is aware of these constraints and is working diligently to refine its framework further, ensuring robust performance in diverse settings.
The Distillation Process: Unlocking Potential
Distillation is an essential technique utilized by DeepSeek, allowing for the transfer of knowledge from larger models to more efficient ones. This strategy not only enhances efficiency but also enables smaller models to outperform others trained on similar data sets. Models like DeepSeek-R1 have shown that even configurations as small as 1.5 billion parameters can achieve competitive results, thereby revolutionizing resource allocation and deployment strategies within AI applications.
The advantages of AI distillation processes are well documented and can be further explored in academic literature, such as this article on model compression techniques at arXiv.
Conclusion: A New Era of AI Reasoning
DeepSeek-R1 signifies a notable advancement in the realm of AI reasoning, challenging established models and setting new performance benchmarks. With its commitment to open-source principles and innovative training methodologies, DeepSeek is well-positioned to lead a new era of AI advancements that prioritize reasoning and adaptability in complex environments.
Embrace the Future of AI with DeepSeek-R1
DeepSeek-R1 represents a groundbreaking stride in artificial intelligence, exemplifying advanced reasoning capabilities through its innovative use of large-scale reinforcement learning and unique training methods. With impressive benchmark results, including an outstanding performance in mathematics and coding, this model not only surpasses established competitors but also signals a shift toward more adaptable and efficient AI solutions. By promoting open-source collaboration, DeepSeek encourages a vibrant community focused on pushing the boundaries of AI technology. To stay informed on the evolution of AI reasoning and the role of open-source technologies, explore further insights on the subject here. Join the conversation and be part of the revolution in AI advancements!