Narrowing the Confidence Gap for Wider AI Adoption
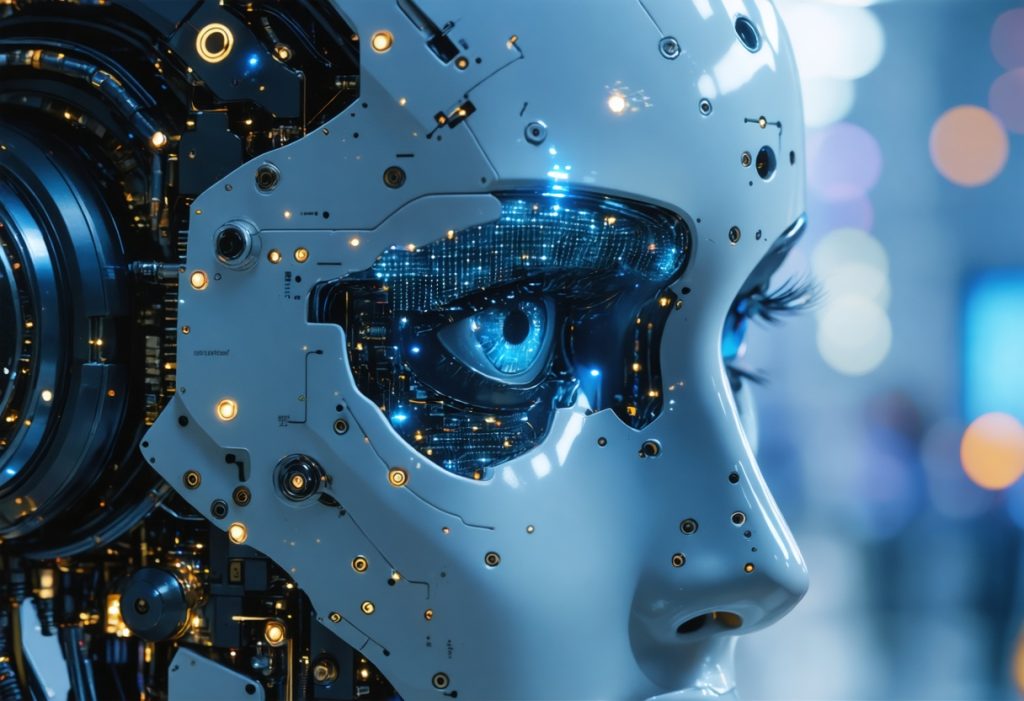
Narrowing the Confidence Gap for Wider AI Adoption
As artificial intelligence continues to revolutionize industries, the initial excitement surrounding its capabilities faces a significant challenge: a growing confidence gap among business leaders. Despite the promise of substantial cost savings—estimated by McKinsey at up to $2.6 trillion across diverse operations—actual implementation lags, with only 20% of generative AI applications making it to production. This disparity between enthusiasm and execution raises critical questions about the barriers to AI adoption.
Challenges such as security concerns, data privacy, compliance risks, and the complexities of managing high-quality data contribute to the hesitance. Furthermore, the lack of transparency and the struggle to demonstrate tangible ROI exacerbate these issues. In this article, we will explore the multifaceted obstacles that organizations face in adopting AI technology and present actionable strategies that business leaders can employ to bridge this confidence gap, ultimately paving the way for a more profound integration of AI across industries.
Understanding the Confidence Gap in AI Adoption
The disparity between the enthusiasm for artificial intelligence and its actual implementation showcases a pronounced confidence gap among business leaders. While many recognize the immense potential for generative AI, the journey from theory to practice is fraught with challenges. To effectively bridge this gap, organizations must understand the underlying issues preventing successful AI adoption and begin strategic interventions that not only mitigate risk but also foster trust amongst stakeholders.
The Importance of High-Quality Data
At the heart of successful AI implementation lies high-quality data. AI models are only as good as the data fed into them, and numerous studies cite poor data quality as a key barrier to AI adoption. According to industry experts, IT professionals often struggle with meeting AI’s demanding data requirements. For AI systems to yield reliable outcomes, organizations must invest in data governance from the ground up. This includes establishing rigorous data quality and integrity controls that ensure the data used for training AI models is accurate and relevant.
Building a Data Governance Framework
A strong data governance framework should encompass data collection, storage, processing, and sharing practices. Organizations need to implement policies that maintain data quality and protect against breaches. By establishing clear roles and responsibilities surrounding data management, organizations can create a culture of accountability and foster confidence in AI initiatives. Regular audits of data practices can further enhance data reliability, making a compelling case for the strategic use of AI.
Ethics and Compliance in AI
The evolving landscape of AI regulations poses both challenges and opportunities for organizations. Many business leaders remain anxious about the legal and ethical implications of deploying AI technologies. The proliferation of regulations necessitates a proactive approach to compliance risks and ethical governance.
Developing Comprehensive Compliance Policies
Creating robust compliance policies that encompass AI technologies is essential. Organizations should establish dedicated AI governance teams responsible for overseeing compliance with legal and ethical standards. Implementing training programs and clear protocols for AI model deployment can help ensure that all AI-powered decisions are made within a framework of accountability, reinforcing trust amongst stakeholders.
Addressing Security and Data Privacy Concerns
Security fears are paramount among businesses that are wary of AI tools. The risks of data exposure and the potential for misuse of AI systems can lead to hesitancy in adoption. As companies increasingly rely on generative AI tools, they must implement security measures to protect sensitive information.
Implementing Robust Data Access Controls
A strategic approach to data access is critical. Organizations should implement strict access controls to prevent unauthorized data usage. By limiting exposure to sensitive data, companies can significantly reduce privacy risks. Employing techniques such as data anonymization or tokenization can further safeguard data while still allowing for meaningful AI analysis.
Enhancing Transparency and Explainability
One of the core issues in AI adoption is the opacity of AI decision-making processes. Many stakeholders are hesitant to trust AI outputs due to the ‘black box’ nature of some AI algorithms. This skepticism can hinder the adoption of AI systems across various sectors.
Boosting AI Explainability
To foster trust, organizations should prioritize transparency. By adopting explainability frameworks, companies can demystify AI outcomes and allow users to understand the rationale behind AI-generated decisions. Tools that provide insights into how models operate and the factors influencing their predictions can significantly enhance user confidence in AI technology.
Defining Clear Business Value
Certain organizations hesitate to invest in AI due to unclear ROI and ambiguous business value. Many leaders regard AI tools as expensive investments with uncertain returns, further contributing to the confidence gap.
Establishing Key Performance Indicators (KPIs)
For organizations to fully leverage AI potential, it is essential to establish clear business objectives and KPIs that demonstrate the value of AI investments. By pinpointing high-impact use cases and focusing on projects that promise swift ROI, businesses can justify their AI initiatives and encourage broader adoption across departments.
Bridging the Skills Gap through Training
A significant barrier to AI adoption is the skills gap that persists in many organizations. While interest in AI is growing, the lack of qualified personnel to lead these initiatives often leads to stagnation. Effective training programs can empower existing employees to utilize AI tools more effectively and increase overall confidence.
Implementing Comprehensive Training Programs
Organizations must prioritize training initiatives to equip employees with the requisite skills to navigate AI technologies. By investing in training programs focused on generative AI and its applications, businesses can mitigate the skills gap that hinders adoption. As employees gain confidence in using AI tools, organizations will likely experience increased innovation and productivity, paving the way for deeper AI integration.
Facilitating Collaborative AI Development
To further close the confidence gap, organizations should foster a culture of collaboration among stakeholders involved in AI development and deployment. Open communication channels that include IT, data scientists, and business leaders can significantly enhance understanding and buy-in for AI initiatives.
Encouraging Cross-Departmental Collaboration
Collaboration encourages knowledge-sharing and enables teams to address concerns regarding AI adoption. By creating cross-functional teams that work together on AI projects, organizations can leverage diverse perspectives and insights, ultimately building a stronger case for AI technologies and turning skepticism into enthusiasm.
Measuring Success and Adapting Strategies
Lastly, organizations should focus on measuring the success of their AI initiatives continually. Assessing the effectiveness of AI systems against set KPIs allows for ongoing adjustments to strategies, ensuring that companies remain agile and responsive to changing market needs.
Continuous Improvement Framework
By establishing a framework for continuous improvement, organizations can stay ahead of challenges while maximizing the potential of AI technologies. Regular evaluations of AI performance and user engagement will result in refined strategies that ensure sustained growth and confidence in AI adoption.
Empowering Organizations for AI Success
In the rapidly evolving landscape of artificial intelligence, bridging the confidence gap is essential for organizations aspiring to harness AI’s transformative potential. By prioritizing high-quality data, developing comprehensive compliance and governance frameworks, addressing security and privacy concerns, enhancing transparency, and demonstrating clear business value, businesses can cultivate an environment conducive to successful AI adoption.
Moreover, investing in training programs and fostering cross-departmental collaboration will equip teams with the skills and confidence needed to innovate and excel in AI initiatives. As organizations adopt a mindset centered on continuous improvement and adaptability, they will not only overcome the hurdles of AI integration but also unlock significant value and competitive advantage.
Ultimately, by embracing these strategies and focusing on cultivating trust among stakeholders, businesses can confidently navigate the complexities of AI technology, leading to wider adoption and a more profound impact across industries. The journey toward AI integration may be challenging, but with the right approach, organizations can turn potential into powerful progress.