Navigating the Data Dilemma: AI’s Promise and Challenge in Financial Services
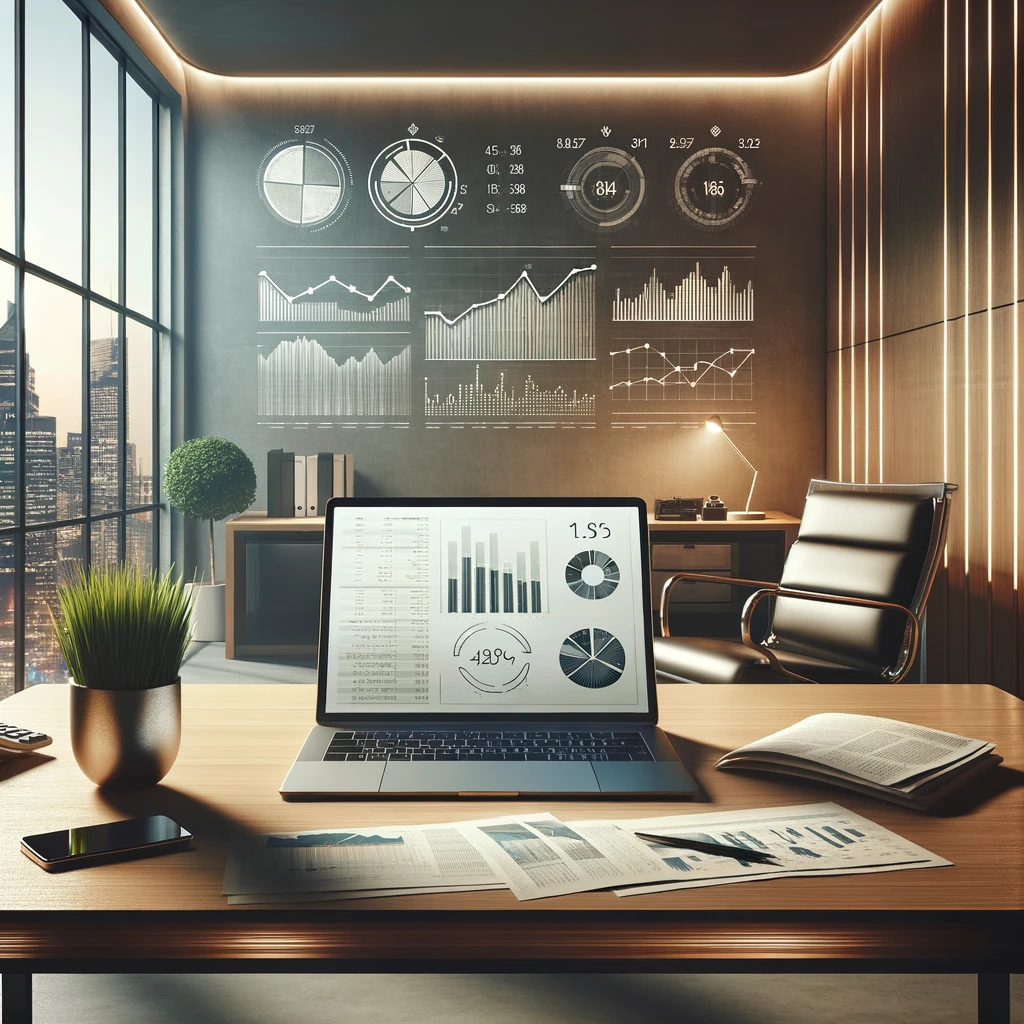
In the rapidly evolving landscape of financial services, the integration of Artificial Intelligence (AI) has become a beacon of transformation, promising unparalleled efficiency and data-driven decision-making. Businesses within the sector are racing to capitalize on AI’s potential, yet many are facing a critical stumbling block: the complexity and quality of underlying data. I’m Ryan Daws, a senior editor with a knack for unearthing the impact of technology on finance, and in this exploration, we’ll delve into how these data issues are putting a wrench in the works.
A recent EXL study reports a staggering 89% of UK insurance and banking firms have adopted AI in the past year, showing commendable initiative. Investments are sky-high, with leaders pouring millions into AI, but there’s a concerning twist—nearly half acknowledge being only “minimally data-driven.” This revelation uncovers a discrepancy that could dilute AI’s efficacy, as an impeccable data infrastructure is non-negotiable for successful AI deployment.
Compounding this dissonance is the novel challenge posed by generative AI, which, despite its buzz, introduces risks such as brand damage and data inaccuracies. It’s not all dire, though. A subset of ‘Strivers’ within the industry demonstrates that a narrow, function-specific AI application can lead to cost efficiency and a competitive edge.
The overarching message is clear: financial services must approach AI with a strategic, data-centric lens. Executives need to ensure that their investments are not just chasing the AI trend but are underpinned by a robust data strategy. Join me as we dissect the implications of this study, unraveling the complexities behind AI’s revolutionary promise and the stubborn data issues obstructing its path to success.
Understanding the AI Integration Challenge in Financial Services
The drive for innovation in financial services has led many firms to adopt Artificial Intelligence (AI) for its potential to revolutionize operations. From automating mundane tasks to providing insights through data analysis, AI stands to redefine the sector. However, the transition is not as straightforward as many envision. The core issue plagots these advancements is data quality and complexity, which, if unaddressed, could significantly impede AI’s transformative impact.
The Data Dilemma: Quality and Complexity
AI thrives on data—the more accurate and comprehensive, the better. For AI systems to work effectively, they require clean, well-organized, and relevant data sets. The existing data infrastructure of many financial institutions is built on legacy systems, which often results in siloed and inconsistent data that can derail the best-intentioned AI initiatives. The dilemma is not just in quantity, but in the quality and complexity of the data being fed into AI models. To leverage the full potential of AI, financial services must invest in their data architecture as much as in AI technology itself.
Generative AI: Innovation Mixed with Caution
Generative AI, a term that’s currently buzz-worthy in the tech community, is a type of AI that can produce content, such as text and images, that can potentially pass for human-generated. While it opens doors to new possibilities in customer interaction and content creation, it also brings substantial risks. Brand damage can occur if generative AI goes unchecked, leading to the propagation of off-brand or erroneous communications. Furthermore, reliance on generative AI without stringent checks can result in decision-making based on inaccurate data. Financial institutions must balance innovation with due diligence to mitigate these risks.
The Strivers’ Approach: Narrow and Strategic AI Use
A group of Strivers within the financial sector has taken a different approach, demonstrating that the strategic, narrowed application of AI can lead to more immediate benefits. By focusing AI deployment on specific functions, these Strivers have been able to attain cost efficiencies and gain a competitive edge. They’ve shown that instead of spreading efforts too thin, concentrating on areas where AI can deliver quick wins allows for a more manageable integration and value demonstration.
The Investment Paradox: Capital Infusion vs. Data Strategy
Despite the large sums poured into AI, the lack of a solid data strategy could make these investments less productive. Financial leaders are often caught between the drive to innovate and the need to uphold rigorous data standards. The EXL study’s findings suggest that the excitement around AI’s capabilities may be obscuring the foundational work required to prepare data systems for such advanced technologies. This paradox highlights the need for a balanced investment strategy, counting data management as a crucial part of the AI implementation budget.
The Role of Leadership in AI Success
For AI deployments to reach their optimal potential, buy-in from the executive board is essential. Leaders need to advocate for a data-centric culture where investments in AI go hand in hand with enhancements to data quality and governance. It is the responsibility of decision-makers to align AI strategies with an overarching vision of data excellence. Without strong leadership paving the way for a strategic, data-focused approach, AI projects in financial services risk falling short of their potential.
In summary, while the integration of AI within financial services heralds a new era of efficiency and innovation, complexity, and quality of underlying data present substantial hurdles. A strategic, focused approach that puts data at the forefront is paramount for overcoming these challenges, and ensuring that AI can deliver on its promise of transformation.
In conclusion, the journey towards harnessing the revolutionary power of Artificial Intelligence in financial services is marked by significant roadblocks, primarily the quality and complexity of data. As we’ve seen, a strategic, data-centric mindset is critical for businesses looking to leverage AI’s full potential. Pioneers in the industry, referred to as Strivers, have illustrated that a concentrated and thoughtful application of AI can yield tangible benefits and a competitive advantage. Leadership plays a pivotal role in driving a culture that values data quality and governance just as much as technological advancement.
For AI to be more than just a fleeting trend in the financial sector, organizations must prioritize a solid data infrastructure. Investments should equally encompass data strategies to ensure the success of AI initiatives. The insights from the EXL study and our discussion underscore the investment paradox where capital infusion without a sound data foundation is a recipe for underperformance.
It is with a clear vision and robust data strategy that financial services can truly embrace AI’s transformative capabilities. Leaders must rise to the occasion, championing data excellence and innovation in equal measure. As companies navigate the complexities of AI integration, those who invest wisely in their data ecosystem will emerge as frontrunners in the financial landscape’s digital transformation. This focus on data will not only empower AI deployment but will also safeguard against the risks associated with emerging technologies such as generative AI.
For financial institutions ready to pave the way, the integration of AI backed by high-quality data holds the promise of unlocking unprecedented efficiency, enhanced customer experiences, and decision-making prowess that will define the future of the industry. Thus, embracing a data-first approach is the key to transforming the promise of AI into a potent reality for financial services.